Webinar: Post Force competition Symposium, where the contestants will showcase their working methods and results
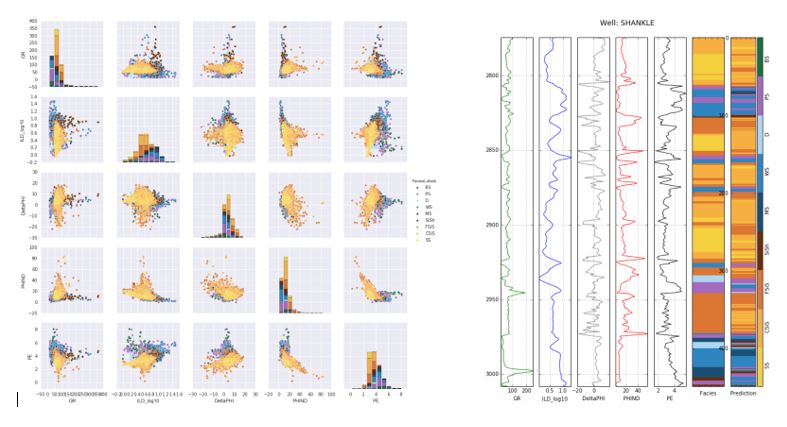
Post Force competition Symposium where the contestants will showcase their working methods and results of their machine learning models for fault mapping and well lithology prediction. We aim to have 6-8 talk of about 20 minutes with ensuing discussion.
Date | Time | Duration | Register by | Location |
28.04.2021 | 15:00 | 6 hours | 27.04.2021 | Teams |
Program
Duration | Start Time Norway | Start Time UTC | Title | Abstract | Author | |
00:10 | 3:00 PM | 1:00 PM | Introduction | Thwe summary of the FORCE 2020 ML on wells and seismic competition | Peter Bormann | |
3:10 PM | 1:10 PM | |||||
1 | 00:20 | 3:10 PM | 1:10 PM | Lots of brilliant ideas ... Too bad they don't work (Predicting Lithology using ML) | Almost all the top scores in the Predicted Lithology competition derive from an accurate use of classic machine learning methodologies and a standard approach to the problem. It is puzzling that more innovative approaches and more sophisticated reasoning do not improve the result in any way. Understanding why this behavior could be very helpful. Here we present and discuss some of the alternative approaches we have tried |
Vincenzo Lipari |
3:30 PM | 1:30 PM | |||||
2 | 00:20 | 3:30 PM | 1:30 PM | Petrophysical guided preconditioning applied to electrofacies classification by machine learning | This work adopted petrophysical perspective to specialize preprocessing to perform missing data imputation, feature selection and engineering using wavelet transform to help differentiate specific lithologies. All the process concentrated on training and testing strategies to fine-tune the classifier. | Lucas Aguiar, Maurício Matos, Jadson Muniz and Rodrigo Canário |
3:50 PM | 1:50 PM | |||||
3 | 00:20 | 3:50 PM | 1:50 PM | Too much data or not enough? | The oil well lithology dataset that we were provided as part of the Predicted Lithology competition contained about 1 million rows of data. However, this data was spread across a mere 100 or so oil wells. Is this too much data or not enough? Let's look to see what we can do to compress the dataset to obtain faster training times while still keeping the benefit of the data that we have. | Campbell Hutcheson |
4:10 PM | 2:10 PM | |||||
4 | 00:20 | 4:10 PM | 2:10 PM | Application of a method of Ensemble learning Lithology Identification | We have performed data preprocessing (standardization, completion of missing data, etc.) for the provided data. We use a variety of machine learning methods (catboost,lightgbm,xgboost) for lithology recognition, and use multiple classification results to vote (stacking) to obtain the final lithology recognition result. | Zhenjia Wang,Qingkai Wang,Yingjian Sun,Zhikai Yang |
4:30 PM | 2:30 PM | |||||
7 | 00:20 | 4:30 PM | 2:30 PM | Stratified CV Fold + regularized gradient boosting approach to lithology prediction | Here we used to a proper validation technique to ensure each change performed on the model reflects a true performance and not a random effect. Hyperparameters were manually tuned to get optimal model performance. | Olawale Ibrahim |
4:50 PM | 2:50 PM | |||||
6 | 00:20 | 4:50 PM | 2:50 PM | Lithology Identification Algorithm using ML | We used a variety of preprocessing methods such as imputation and scaling the data. We compared the performance of each of well logs, then select multiple combination of well logs as the input. We used XGBoost and LightGBM as the training algorithms, and tuning the hyperparameters to achieve the best result. | Ridwan Iyas |
5:10 PM | 3:10 PM | |||||
5 | 00:20 | 5:10 PM | 3:10 PM | Fault identification Algorithm: FaultSAM | This study presents our contribution in supporting the exploration stage, especially in fault interpretations. In generating the fault model, we used Neural Network to accommodate the learning process for enabling the machine to work with 2D image data and detecting the objects. We had to get through 5 stages, namely: pre – processing, processing, evaluation and validation, post - processing, and output. | Marcelinus Febbi and Ridho Dinartho |
5:30 PM | 3:30 PM | |||||
8 | 00:20 | 5:30 PM | 3:30 PM | Fault interpretation from seismic using a 3D CNN trained on synthetic models. | We used a 3D Convolutional Neural Net to solve the problem. We found that the results were improved by augmentation of the training data and by adding noise and altering the frequency of the synthetics. The resulting CNN was fast and effective, with best results for the smaller faults. | Margaux Ledieu, Paul Gillespie (Equinor) |
5:50 PM | 3:50 PM | |||||
9 | 00:20 | 5:50 PM | 3:50 PM | Lithology Prediction by using Machine and Deep Learning Algorithms | Different machine learning method were tried to classify lithofacies, in addition a fully conected neural netwok was constructed and optimized based on a bayesian approach. The presentation will explore how the best ML algorithms perfom compared with a Neural Network in a classification problem. | John Masapanta, Supervisor: Arild Buland (Equinor) |
6:10 PM | 4:10 PM | |||||
11 | 00:20 | 6:10 PM | 4:10 PM | My experiences doing ML with well logs | In the world of data, it's often interesting to see the creative and advanced approaches to solving a machine learning problem, but what if you aren't an expert? This presentation goes through the challenges of a beginner who had no prior experience in machine learning go through the steps and curiosities of coming up with a reasonable solution. This will be great for those who are just getting started and want to learn or revisit what it was like doing ML for the first time. | Jeremy Zhao |
6:30 PM | 4:30 PM | |||||
10 | 00:20 | 6:30 PM | 4:30 PM | Prediction of lithofacies based on wavelet transformation and machine learning algorithm | In this work, we study the evaluation of lithofacies values using the machine learning algorithms in the determination of classification from various well log data. We also use the wavelet-transformed data in machine learning algorithms to identify geological properties from the well log data. We have compared the the machine learning algorithms including KNN, Decision Tree, Random Forest, XGBoost, and LightGBM. The evaluation of the model score is conducted by using metrics such as accuracy, Hamming loss, and penalty matrix. In addition, the influence of the dataset features on the prediction is investigated using the machine learning algorithms. | Timur |
6:50 PM | 4:50 PM |
How a webinar works
You register as usual through the registration button above. Once you are registered you will get an invitation via email to join this webinar.
FORCE uses Teams Video for this webinar, and has proven to work successfully.
We recomment that everyone joining turn off their camera and microphone when joining.
If you have any questions you can use the chat or wait until the end of the talk.
FORCE seminars have previously been fully booked with waiting lists so you are encouraged to sign up as soon as you know you will attend.
Participation fees:
FORCE members: Free
Non-members: NOK 350,-
University/student: Free
Important information:
You can register as a FORCE member and pay "FORCE member" price if you are an employee of a member company.
All FORCE member companies are listed here.
Payment is made online by credit card. Please note that no refunds will be given after you have signed up.
If you for any reason can not attend the workshop, you are welcome to send a representative, just inform Linn Smerud as soon as there are changes.
If you have any questions please contact Linn Smerud at the FORCE secretariat.
Updated: 29/04/2021